How to Build a Machine Learning Portfolio
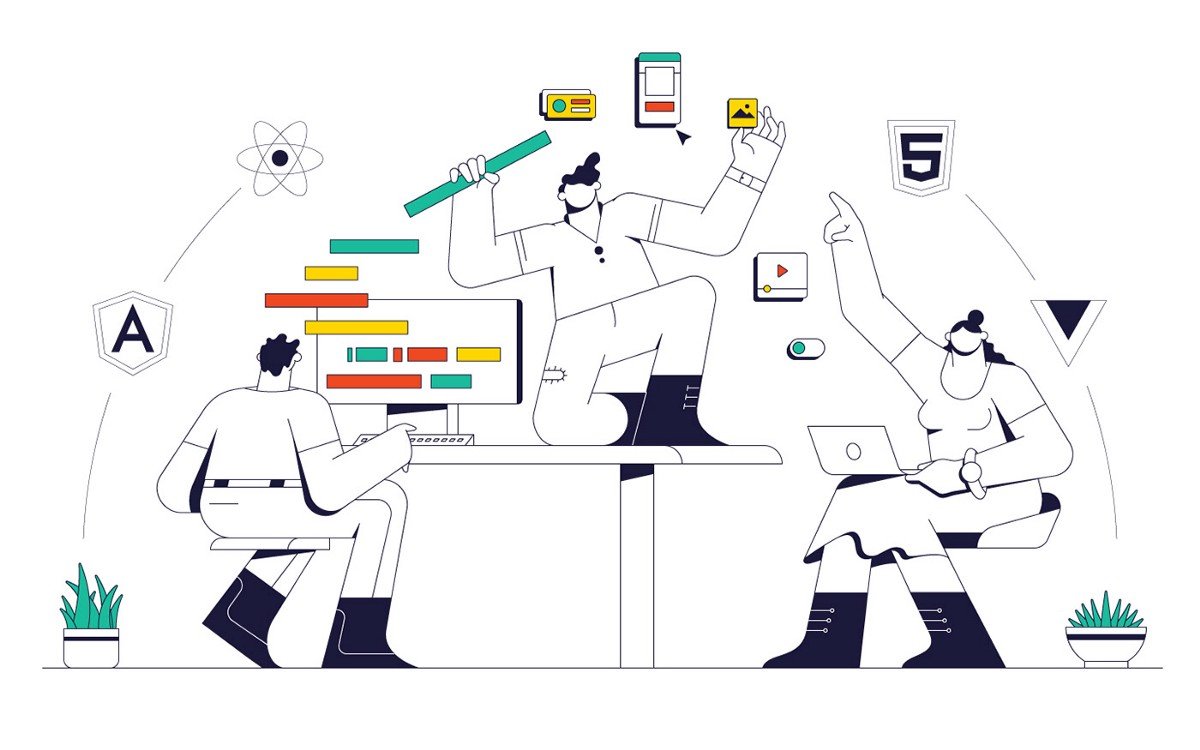
The most important thing that every machine learning enthusiast needs to have is a portfolio. Not having a portfolio in this industry is like driving a car without a license. You may be able to get far, but as soon as someone notices, that’s it. A portfolio is essential for a ton of different types of careers. But, a machine learning enthusiast is in a unique position since their credibility as a problem solver entirely relies on their portfolio. Every employer is going to look at their past projects and determine whether they even deserve an interview. So, in a way, your portfolio is the key that will open the door to machine learning jobs. The first step would be to take any Machine Learning Certification Course to get hands-on experience and learn directly from experts would make it easier for making a good Portfolio. But, how exactly can you build a portfolio that stands out? How can you build a portfolio that attracts employers? Let’s answer those questions right now.
Table of Contents
Make Your Portfolio Based on Real-Time Use Cases
One of the mistakes that young machine learning experts. They make it that they don’t pick the right projects to work on. You’re not supposed to go and see why Baltimore won the 1971 Super Bowl and what Dallas could’ve done differently to win the game. That’s not research that can benefit anyone. Instead, you can work on modern, global situations. A great example of that would be COVID-19. Such an unpredictable situation could use some research to make it slightly more predictable. So, make projects on use cases similar to this. Employers value your results, especially when they have the potential to help humanity in general. Another example would be the implementation of machine learning in Africa to help the locals deal with the current locust problem. By using machine learning algorithms, it’s possible to determine the paths of the locusts and warn farmers months in advance.
The Journey Matters Just as Much as the Conclusion
A lot of people will tell you that machine learning is all about the final answer. The actionable conclusion is what companies can use to make changes to their process and formulate a more stable and efficient methodology. However, we’re here to tell that even though the answer is important, how you got to that answer holds its own significance as well. If your process isn’t viable and isn’t documented appropriately, your findings may not hold much ground. It doesn’t matter how groundbreaking your conclusion is. You have to make sure that your journey to that conclusion is 100% legit.

Think Outside the Box
Another common mistake a lot of folks make is that they don’t think outside the box. There’s nothing wrong with having a by-the-book mentality in life. But, when it comes to machine learning, filling your portfolio with cliché ML projects is going to reduce the chances of you getting hired. For instance, think about a project like spam detection. It sounds like a pretty neat idea that’s really helpful in this day and age. But, you have to think about the fact that literally every student studying machine learning has made some variation of this project. There’s a lot of other projects similar to this that might feel like a promising addition to your portfolio, but they won’t be adding much to your credentials. Instead, think of outside-the-box projects. Do some research and take a look at sample projects. Think about how you can make them different and unique. If your portfolio has diverse practical ML projects that the rest do not, you’re good to go.
Refine Your Solutions
There are a hundred ways to drive to work every day. But which route will you take? You’re not going to take the first route you find if it adds 20 minutes to your commute. That just doesn’t sound like an efficient thing to do. But why are you using the same strategy in your machine learning projects? Machine learning is an industry that doesn’t need a solution to a problem. Anyone can come to a solution. If that was the only task at hand, there wouldn’t be any difference between a good and mediocre data scientist. Your job is to find the most efficient solution to a problem. When you present a portfolio with sound conclusions, the first thing any employer will try to think is if there is a better way to solve that problem. If there is and you didn’t think of it (or worse, didn’t think it was important enough to implement), that’s a huge red flag for employers looking to hire people.
Final Thoughts
To sum up the conversation, it’s important to remember that a machine learning portfolio is the only thing that’s going to get you accepted for your dream job. And, if the portfolio doesn’t contain some of the points that we’ve discussed throughout this article, it’s not really going to push your career further. If you are interested in the packaging of Machinery then check this: Custom Rigid Boxes
If you are interested in making an ML project check this: Machine Learning Projects Ideas for Beginners